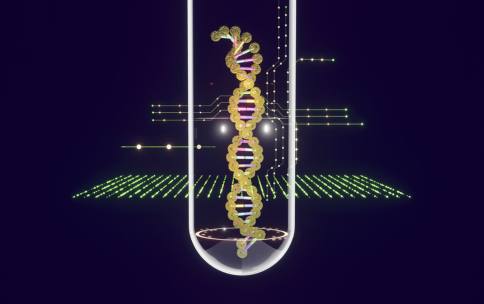
To advance our understanding of medicine and disease, researchers often collect a large amount of data related to patient health for study and analysis. After data is collected, researchers may reuse the data to address new questions about human health. However, given that researchers collect large datasets using different methods and standards, combining and analyzing them is inherently challenging. One solution to this problem is to generate a knowledge graph, which is a structured data model that can be used to analyze, interpret, and identify relationships between multiple datasets.
The NIH Common Fund Data Ecosystem (CFDE) aims to accelerate discovery through the broad use of data generated across multiple Common Fund programs. Deanne Taylor, Ph.D., a CFDE awardee, worked with colleagues from two Common Fund programs, Gabriella Miller Kids First Pediatric Research (Kids First) and the Human BioMolecular Atlas Program (HuBMAP), to create Petagraph. Petagraph is a publicly available knowledge graph that allows users to integrate and analyze datasets collected by multiple researchers.
Petagraph combines and standardizes varied data types, such as clinical information and data about proteins, genes, and cells, so that researchers can analyze different data together. Petagraph connects measurable or observable traits, such as height or eye color, with genetic information, and can be used to identify genes or cell types linked to certain diseases. These features help researchers to efficiently tackle emerging medical issues like identifying new drugs or new uses for existing medications. The current version of Petagraph supports data from ten different research programs, but its design is both flexible and scalable so new data can be added to it, enhancing its usefulness. Petagraph’s storage requirements are minimal, and it can be freely downloaded onto a laptop.
Dr. Taylor and colleagues have published an article describing the steps used to create Petagraph, highlighting the inclusion of a comprehensive data dictionary, and instructions on how to add more data to create a customized knowledge graph. Several use cases demonstrate how Petagraph can be used to identify relationships between genes and particular health conditions, understand potential medical side effects, and identify gene targets for future research.
Much work and careful consideration is needed for Petagraph to reach its full potential. The data will need to be continuously refined; standards will need to be developed to minimize bias as well as ensuring transparency, and scalability will need to be maintained. While work remains ongoing, Petagraph demonstrates the potential for researchers to use data across multiple biomedical datasets to further scientific discovery.
Reference: Stear BJ, Mohseni Ahooyi T, Simmons JA, Kollar C, Hartman L, Beigel K, Lahiri A, Vasisht S, Callahan TJ, Nemarich CM, Silverstein JC, Taylor DM. Petagraph: A large-scale unifying knowledge graph framework for integrating biomolecular and biomedical data. Sci Data. 2024 Dec 18;11(1):1338. doi: 10.1038/s41597-024-04070-w. PMID: 39695169; PMCID: PMC11655564.