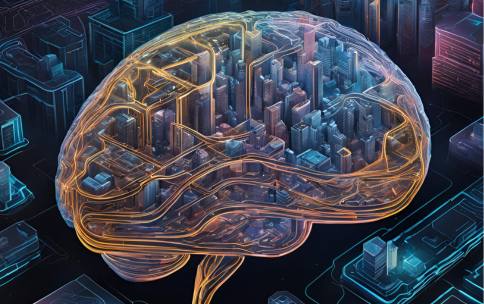
The field of artificial intelligence (AI) and machine learning (ML) in the biomedical sciences is moving at an unprecedented rate. AI/ML models can process vast amounts of medical data that may prove critical to the future of medical decision making. But do human biases influence these models? In a new pilot project from the Patient-Focused Collaborative Hospital Repository Uniting Standards (CHoRUS) for Equitable AI, researchers found the AI/ML models may be susceptible to social biases and warned that researchers should be aware of these biases and their influence on decision making. CHoRUS is part of the NIH Common Fund Bridge to Artificial Intelligence (Bridge2AI) program, which aims to generate tools, resources, and richly detailed data that are responsive to AI approaches.
To better understand the impact these biases may have on future AI/ML model predictions, Eric Rosenthal, M.D., a neurologist at Massachusetts General Hospital, led a team of CHoRUS researchers in a review of available electronic health record (EHR) data from two neuroscience intensive care units (ICUs) to understand how different factors may change decision making outcomes for life-sustaining therapy (LST). Life-sustaining therapies are treatments that prolong human life (e.g., mechanical ventilation) but do not necessarily cure a condition. The research team focused on how LST decisions changed based on different social determinants of health (SDOHs), which are the social, physical, and economic conditions where people are born, grow, live, work, age, and play that influence a persons’ health and overall wellbeing. According to Dr. Rosenthal and his team, this is the first study of this size to examine the association between SDOH and LST.
The study team analyzed seven years of clinical data across eight individual level SDOH variables (age, ethnicity, race, sex, educational attainment, primary language, insurance, and marital status). The study team also assessed neighborhood-level SDOH, or where a person lives, to understand potential community factors on decision making. In their analysis, the team observed that individual-level SDOH factors were more likely to predict whether a patience received LSTs even when compared to other admission factors, such as condition severity at ICU admission. While the impact of SDOH’s on life sustaining therapy predictions is significant, this study only analyzed data from an urban area in a single state. Furthermore, patients who did not have a listed address were excluded from the analysis, so more work will need to be done to better understand model predictions for those without a permanent living situation.
According to the authors, the study highlights intriguing connections on how SDOH may shape shared decision-making in neuroscience intensive care unit settings. They also issued a warning that the AI/ML models are susceptible to biases related to SDOH, and that controlling for SDOH may be an important factor to avoid encoding bias into these models.
Read more:
Kwak GH, Kamdar HA, Douglas MJ, Hu H, Ack SE, Lissak IA, Williams AE, Yechoor N, Rosenthal ES. Social Determinants of Health and Limitation of Life-Sustaining Therapy in Neurocritical Care: A CHoRUS Pilot Project. Neurocrit Care. 2024 Jun 6. doi: 10.1007/s12028-024-02007-0. Epub ahead of print. PMID: 38844599.
Reference:
Social Determinants of Health (SDOH). U.S. Centers for Disease Control and Prevention (CDC), January 17, 2024.